In the fast-paced realm of artificial intelligence (AI), the development of advanced hardware has been a critical driving force. As AI algorithms become more intricate and demanding, traditional computing systems are struggling to keep up with the increasing complexity of tasks. This has prompted the exploration of quantum computing and neuromorphic chips, offering unprecedented AI revolution opportunities.
The Quantum Leap: Quantum Computing’s Potential for AI : AI Hardware
Quantum computing stands as a beacon of hope for solving complex problems that were previously deemed insurmountable due to the limitations of classical computers. Unlike classical bits, qubits can exist in multiple states simultaneously due to superposition and entanglement principles. This parallelism could revolutionize AI by speeding up tasks such as optimization, cryptography, and machine learning.
Quantum computing’s most significant impact on AI lies in its ability to accelerate certain AI algorithms. Machine learning processes that depend heavily on optimization, such as training deep neural networks, could witness a remarkable speedup. Quantum annealing, a quantum optimization technique, can be applied to enhance the training process by efficiently searching through complex parameter spaces, facilitating quicker convergence to optimal solutions.
Another realm where quantum computing showcases its prowess is in enhancing data analysis. With the ability to process vast datasets more efficiently, quantum computers could unravel hidden patterns and correlations that classical computers might overlook. This significantly affects AI applications like personalized medicine and financial modeling, where precise analysis of extensive data is vital.
Nonetheless, the road to realizing the potential of quantum computing for AI is fraught with challenges. Quantum systems are exceptionally delicate and susceptible to errors caused by decoherence. Building and maintaining stable quantum hardware is a formidable task that requires innovative error correction techniques. Moreover, programming quantum computers demands a shift in the mindset of AI researchers and developers, as the algorithms and languages used will significantly differ from those of classical computing.
Neuromorphic Chips: Emulating the Human Brain
Quantum computing aims to boost AI’s computational power, while neuromorphic computing draws from the human brain to transform information processing. Neuromorphic chips, also known as brain-inspired chips, emulate the parallel and energy-efficient nature of the human brain’s neural networks. Unlike traditional von Neumann architecture, where data processing and memory storage are separate, neuromorphic chips merge these functions, mirroring the brain’s efficient information processing.
One of the most significant advantages of neuromorphic computing is its potential for ultra-low power consumption. Traditional AI algorithms, particularly deep learning, are notorious for their high energy demands. Neuromorphic chips (AI Hardware), on the other hand, leverage the brain’s ability to process information with minimal energy expenditure. This makes them ideal candidates for edge computing, enabling AI systems to be embedded in devices with limited power resources, such as sensors and IoT devices.
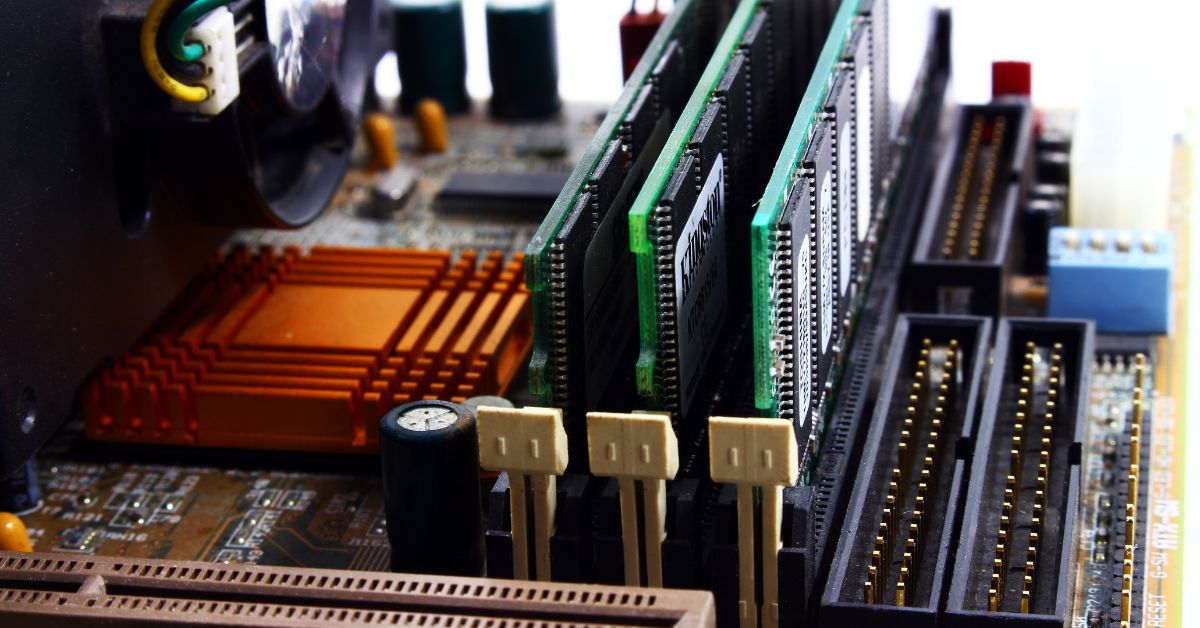
Moreover, neuromorphic chips excel in certain AI tasks that align with their architecture’s strengths. They are exceptionally adept at tasks requiring pattern recognition, such as image and speech recognition. The inherent parallelism and adaptability of neuromorphic systems lend themselves well to these applications.
However, neuromorphic computing also faces its set of challenges. The AI Hardware and software ecosystems for neuromorphic chips are still in their nascent stages, limiting the availability of resources for researchers and developers. Additionally, designing algorithms that can effectively harness the unique capabilities of these chips remains an ongoing research area.
Synergy and Future Prospects : AI Hardware
The worlds of quantum computing and neuromorphic computing might seem disparate, but their convergence could offer synergistic benefits to AI. Quantum computers could potentially help in training complex neuromorphic networks more efficiently by speeding up optimization processes. In turn, neuromorphic chips (AI Hardware) could provide a platform for quantum error correction, aiding in the stability and reliability of quantum computations.
The future prospects of AI hardware evolution hold promise but require a comprehensive approach. Researchers must work collaboratively to bridge the gaps between theoretical potential and practical implementation. Interdisciplinary collaboration is key to addressing challenges in quantum noise, error correction, programming paradigms, and neuromorphic architecture scalability.
As the field continues to advance, stakeholders must also consider the ethical implications of these technologies. Quantum computing’s potential to disrupt current encryption methods raises concerns about data security and privacy. Neuromorphic computing’s ability to mimic human-like processing could push the boundaries of AI ethics, necessitating responsible development and deployment guidelines.
In conclusion, the evolution of AI hardware has reached a pivotal juncture with the emergence of quantum computing and neuromorphic chips. Quantum computing’s unparalleled computational power and neuromorphic computing’s brain-inspired efficiency are poised to redefine the capabilities of AI systems. The future promises exciting possibilities, but their realization demands collaborative efforts, robust research, and ethical considerations. As these technologies continue to mature, we stand on the brink of an AI revolution that could reshape industries and societies in ways we are only beginning to comprehend.
Read This Now – AI-Powered Agriculture: Improving Crop Yield and Sustainable Farming Practices