Artificial Intelligence (AI) has transformed the world, revolutionizing various industries, from healthcare and finance to transportation and entertainment. However, as AI systems become increasingly prevalent, there is growing concern about their potential biases and unfairness. Ensuring that AI remains fair and unbiased presents a complex set of challenges, as the technology is only as good as the data it learns from and the algorithms it employs. This article explores the key challenges faced in achieving fairness and impartiality in artificial intelligence systems.
Biased Data
One of the primary challenges in ensuring AI fairness is biased data. artificial intelligence algorithms learn patterns and make decisions based on the data they are trained on. If the data is biased, the artificial intelligence model will also learn and perpetuate those biases. Biases can emerge from historical human decisions, prejudices, or skewed sample selection. For instance, a facial recognition system may perform poorly on individuals of certain ethnicities if the training data predominantly consists of one racial group.
AI Algorithmic Bias
Beyond biased data, AI models can also inherit biases from the algorithms themselves. Some algorithms may inadvertently favor specific groups or demographics due to the way they process information or optimize for certain outcomes. Algorithmic bias can be challenging to detect and rectify, as it often requires a deep understanding of complex models and their interactions with data.
Lack of Diversity in Development
A lack of diversity in the development teams can also contribute to biased AI. If artificial intelligence teams lack representation from different backgrounds, experiences, and perspectives, there is a higher chance of overlooking potential biases during the development process. Diverse teams can bring varied insights that help identify and address bias more effectively.
AI Explainability and Interpretability
AI models are becoming increasingly sophisticated and complex, making it difficult to understand how they arrive at their decisions. The lack of explainability and interpretability can be problematic when artificial intelligence systems are employed in critical areas such as healthcare or legal decisions. Understanding the logic behind artificial intelligence decisions is essential for detecting and rectifying bias.
Constantly Evolving AI Bias
AI systems are not static; they continue to learn and adapt from new data. This dynamic nature means that biases may arise or evolve over time, even in previously unbiased artificial intelligence models. Monitoring and continuously updating artificial intelligence systems to ensure fairness is an ongoing challenge.
Trade-offs Between Accuracy and Fairness
Striking a balance between accuracy and fairness can be a significant challenge. Some fairness interventions may reduce overall performance, leading to a trade-off between fairness and accuracy. Developers must carefully consider how to optimize their models to avoid exacerbating existing biases or introducing new ones.
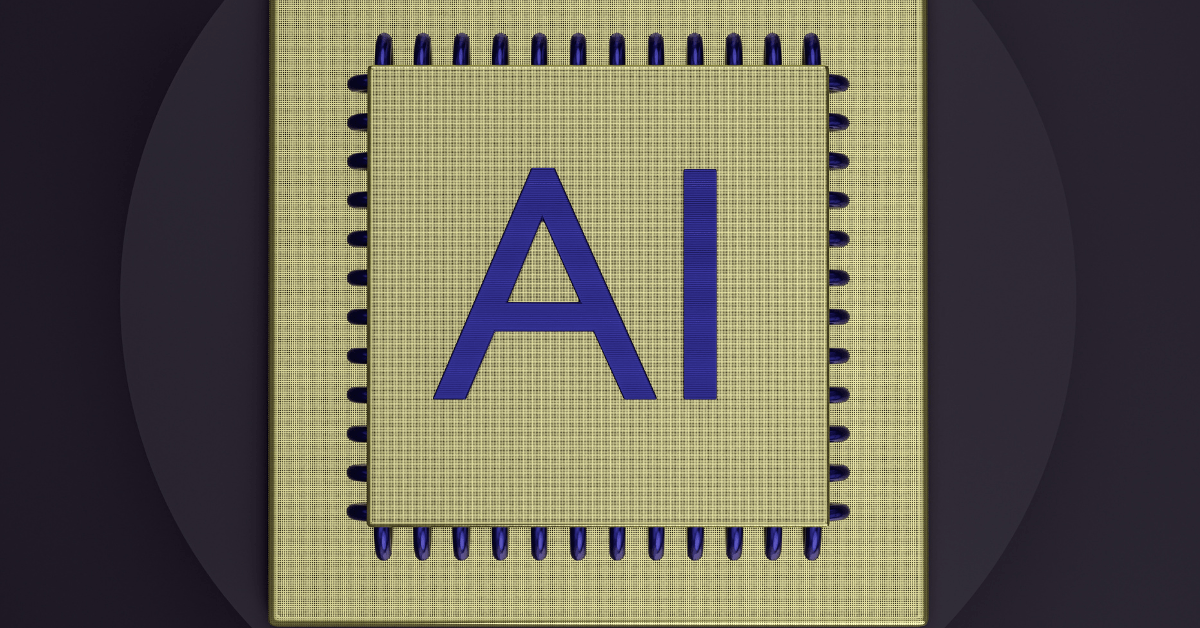
Preemptive Bias Detection
Detecting bias in artificial intelligence systems before deployment is essential to prevent potential harm. However, preemptive bias detection can be difficult, especially when AI models are highly complex. Rigorous testing and evaluation frameworks are necessary to identify and address bias in the early stages of development.
Data Privacy Concerns
Addressing bias in AI sometimes requires access to sensitive data, such as demographic information. Balancing the need for data privacy with the need for transparency in artificial intelligence decision-making poses a delicate challenge. Striking the right balance ensures fair AI systems without compromising user privacy.
Legal and Ethical Considerations
AI systems have the potential to impact individuals and communities significantly. This raises important legal and ethical questions about accountability, responsibility, and transparency. Establishing clear guidelines and regulations to govern artificial intelligence development and deployment is crucial to safeguard against bias and ensure fairness.
The challenges of ensuring that AI is fair and unbiased are multifaceted and demand thoughtful solutions from developers, researchers, and policymakers. Biased data, algorithmic bias, lack of diversity in development teams, and constant evolution of bias are some of the key challenges that need to be addressed. Maintaining a fair and equitable AI ecosystem requires a collaborative effort from all stakeholders to build transparent, accountable, and inclusive AI systems that benefit society as a whole. By recognizing and tackling these challenges, we can unlock the true potential of artificial intelligence while upholding the principles of fairness and equality.
Read This Now – The Impact of AI on the Future of Work
Hi sparerun.com administrator, You always provide practical solutions and recommendations.
Thank you so much for your valuable feedback.