The integration of artificial intelligence (AI) into various sectors has transformed industries and streamlined processes, and the judicial system is no exception. The emergence of AI in judicial systems has brought about significant advancements, especially in predictive legal analytics. This innovative application of AI has the potential to revolutionize the way legal professionals approach case management, decision-making, and the administration of justice. However, with its promise come a set of challenges that need careful consideration. In this article, we delve into the prospects and challenges of AI in judicial systems, focusing on the paradigm of predictive legal analytics.
Prospects of Predictive Legal Analytics:
Enhanced Decision-Making: AI in Judicial Systems
One of the key prospects of AI in judicial systems is the ability to enhance decision-making. Predictive legal analytics involves the use of AI algorithms to analyze vast volumes of legal data, including historical case outcomes, judge decisions, and legal precedents. This enables legal professionals to make more informed decisions by predicting the potential outcomes of cases based on patterns and trends extracted from the data.
Reduced Backlog:
Overloaded court dockets and case backlogs are perennial challenges faced by judicial systems. Predictive legal analytics can aid in prioritizing cases based on their likelihood of settlement, thereby helping courts manage their schedules more efficiently. By identifying cases that have a higher probability of resolution, courts can allocate resources effectively and reduce delays in the legal process.
Fairness and Consistency:
AI algorithms are designed to be impartial, thus minimizing the potential for human bias in decision-making. Predictive legal analytics can assist in ensuring fairness and consistency in judgments by analyzing patterns of previous rulings and detecting any disparities. This could lead to a more equitable administration of justice.
Resource Optimization:
Legal professionals spend a significant amount of time conducting legal research and preparing cases. AI-powered tools can expedite this process by automating research tasks, thus enabling lawyers to focus more on strategic aspects of their cases. This, in turn, can lead to improved efficiency and productivity within the legal profession.
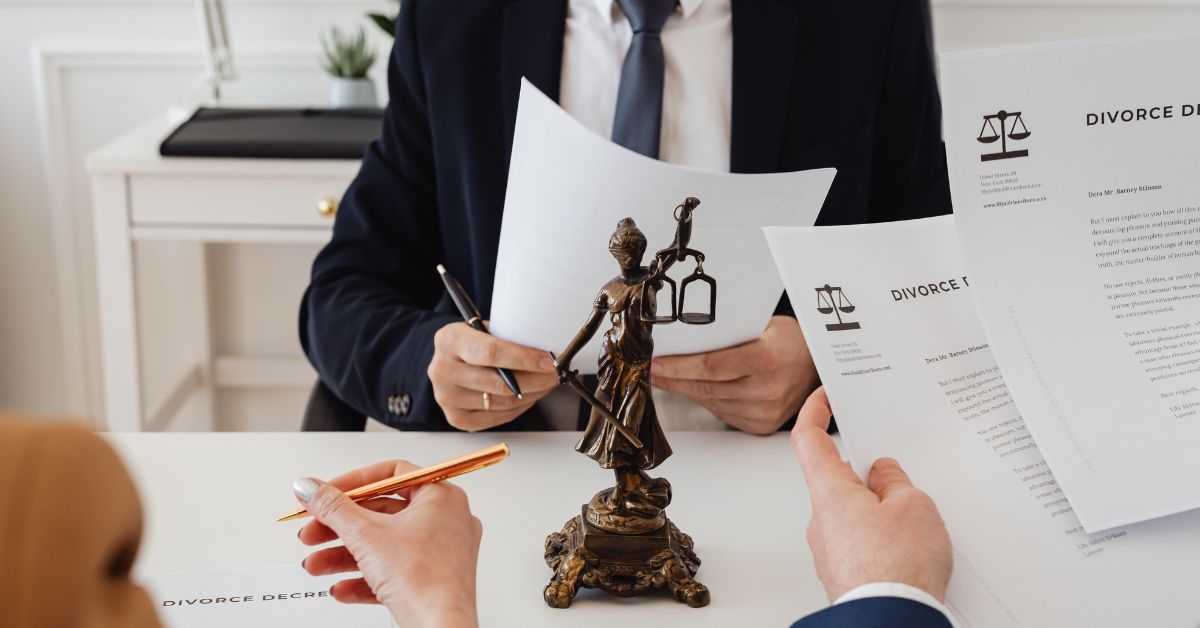
Challenges of Predictive Legal Analytics:
Data Quality and Bias: AI in Judicial Systems
The accuracy of AI predictions depends heavily on the quality and diversity of the training data. If historical legal data is biased or incomplete, the predictions made by AI algorithms could inherit those biases. Ensuring representative and comprehensive data is crucial to avoid perpetuating systemic biases in the judicial system.
Transparency and Interpretability:
AI algorithms used in predictive legal analytics are often complex and difficult to interpret. This lack of transparency can undermine trust in the technology, as legal professionals may be hesitant to rely on predictions that they cannot fully understand. Developing methods to make AI decisions more transparent and interpretable is a pressing challenge.
Ethical Concerns:
The use of AI in judicial systems raises ethical questions about the role of technology in decision-making processes that have far-reaching consequences for individuals’ lives. Guidelines and regulations are essential to ensure responsible and ethical AI use within the legal context.
Loss of Human Judgment:
While AI can provide valuable insights, it cannot replace the nuanced and empathetic judgment of experienced legal professionals. Over-reliance on AI predictions might lead to a diminution of the human element in the legal process, which could have unintended consequences for justice administration.
Striking a Balance: AI in Judicial Systems
To harness the full potential of predictive legal analytics while addressing its challenges, a balanced approach is essential. Here are some strategies to consider:
Quality Data Collection:
Ensuring that the training data used for AI algorithms is diverse, comprehensive, and free from biases is fundamental. Collaborations between legal experts and data scientists can help curate datasets that are more representative of real-world legal scenarios.
Interpretable AI: AI in Judicial Systems
Developing AI models that provide explanations for their predictions can foster trust and understanding among legal professionals. Techniques such as interpretable machine learning and model visualization can help make AI decisions more transparent.
Human-AI Collaboration:
AI should be seen as a tool that complements human expertise rather than a replacement. Legal professionals can integrate AI-generated predictions into their decision-making, merging technology advantages with experienced legal judgment.
Ethical Frameworks: AI in Judicial Systems
Creating ethical guidelines and regulations for AI in judicial systems is crucial for ensuring fairness, accountability, and transparency. This includes regular audits of AI systems, data privacy protection, and ongoing monitoring for biases.
AI integration in judicial systems, especially predictive legal analytics, promises to improve decision-making, reduce backlog, and ensure fairness. However, the challenges of data quality, bias, transparency, and ethics cannot be ignored. Balancing AI, human judgment, and ethics will be key to reaping AI’s benefits while preserving justice. As technology evolves, judicious AI implementation can enhance efficiency, fairness, and equity in the legal system.
Read This Now – AI in Banking: Revolutionizing Banking, Investment, and Fraud Detection