The rapid evolution of technology has brought about immense convenience and opportunities, but it has also given rise to an alarming surge in cyber threats. Cybercriminals constantly devise new and sophisticated ways to breach security systems, making traditional cybersecurity methods inadequate. In this increasingly complex landscape, artificial intelligence (AI) has emerged as a powerful tool to fortify our defenses against evolving cyber threats. Specifically, machine learning (ML) is at the forefront of this revolution, enabling proactive and adaptive security measures that can stay one step ahead of cyber adversaries.
The Growing Complexity of Cyber Threats
Modern cyber threats are no longer confined to simple viruses or malware. The contemporary threat landscape encompasses a wide array of challenges, such as advanced persistent threats (APTs), ransomware attacks, zero-day exploits, and phishing scams. These threats are highly dynamic, capable of morphing and adapting to changing circumstances, rendering static rule-based security systems ineffective.
This complexity demands a paradigm shift in cybersecurity strategies. It requires security solutions that can learn from historical and real-time data, adapt to new attack patterns, and swiftly respond to emerging threats. This is precisely where AI and machine learning come into play.
Harnessing Machine Learning for Cybersecurity
Machine learning, a subset of AI, empowers computers to learn from data and improve their performance over time without being explicitly programmed. It holds immense potential for cybersecurity, as it can process massive amounts of data, detect patterns, and make intelligent decisions in real time. Here’s how machine learning is revolutionizing cybersecurity:
Threat Detection and Prevention: AI in Cybersecurity
Traditional signature-based detection methods are reactive and struggle to identify previously unseen threats. Machine learning algorithms can analyze vast datasets to identify anomalies, recognize patterns, and establish a baseline of normal behavior. When deviations occur, these algorithms can raise alerts, enabling security teams to intervene before a breach occurs.
Behavioral Analysis: AI in Cybersecurity
Machine learning models excel at behavioral analysis. By monitoring users’ and systems’ behavior, these models can identify unusual actions that may indicate a cyber attack. For instance, if an employee suddenly starts accessing sensitive data they’ve never accessed before, the system can flag this as suspicious behavior.
Advanced Malware Detection: AI in Cybersecurity
Modern malware is often polymorphic, altering its code to evade detection. Machine learning algorithms can analyze code and behavior patterns to identify malware variants, even if they have never been encountered before.
Phishing and Fraud Detection: AI in Cybersecurity
Phishing attacks are a major concern for organizations and individuals alike. Machine learning models can analyze email content, sender behavior, and other contextual information to determine the likelihood of an email being a phishing attempt.
Real-time Response: AI in Cybersecurity
ML-powered systems can respond to threats in real time. For example, if a system detects unauthorized access, it can immediately take action to block the intruder and prevent further damage.
Reducing False Positives: AI in Cybersecurity
One challenge in cybersecurity is dealing with an overwhelming number of false positives, which can desensitize security teams to actual threats. Machine learning algorithms can refine their detection criteria over time, reducing false positives and allowing security professionals to focus on genuine threats.
Vulnerability Management: AI in Cybersecurity
Machine learning can help identify vulnerabilities in a system or application by analyzing code and configuration. This proactive approach allows organizations to patch vulnerabilities before they are exploited by cybercriminals.
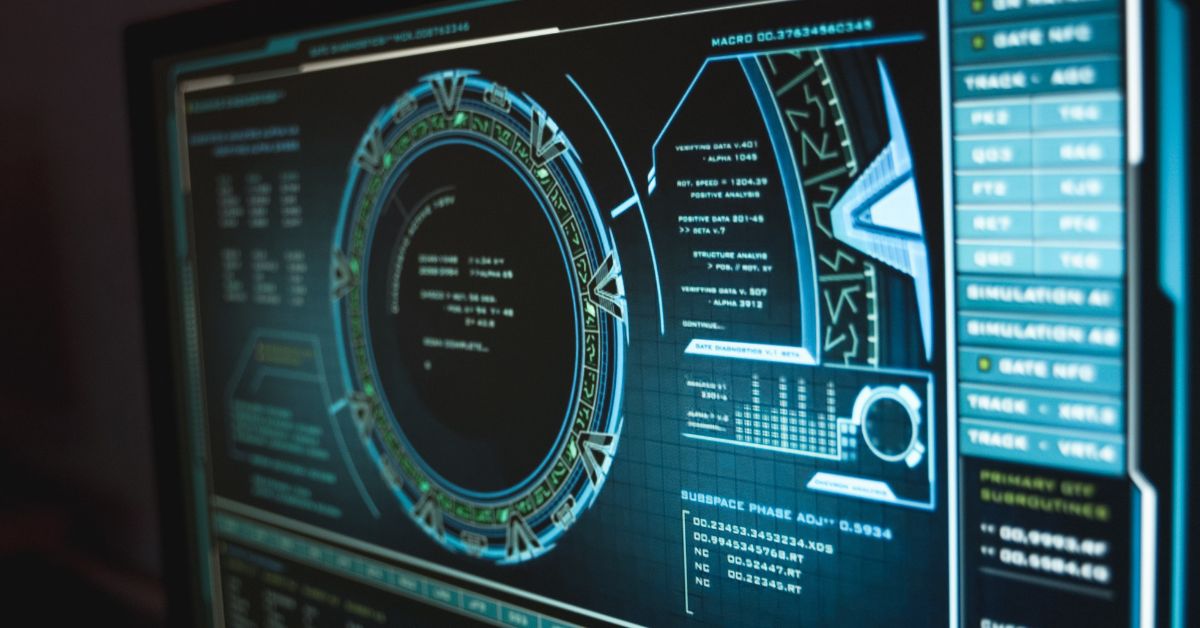
Challenges and Future Directions
While machine learning holds immense promise for bolstering cybersecurity, it’s not without its challenges:
Data Quality and Quantity:
ML algorithms require substantial amounts of high-quality data for training. Gathering and labeling this data can be a resource-intensive process.
Adversarial Attacks:
Cybercriminals can deploy adversarial attacks to manipulate machine learning models, causing them to misclassify data. Researchers are actively working on creating robust models that can withstand such attacks.
Model Interpretability:
As machine learning models become more complex, understanding how they arrive at their decisions becomes increasingly difficult. Ensuring transparency and interpretability is crucial, especially in security-sensitive applications.
Privacy Concerns:
Analyzing sensitive data to train machine learning models can raise privacy concerns. Striking a balance between effective cybersecurity and user privacy is essential.
The future of AI in cybersecurity looks promising:
Zero-Day Threat Detection:
Machine learning can play a pivotal role in identifying and mitigating zero-day threats by recognizing unusual behaviors and attack patterns that haven’t been seen before.
Autonomous Cyber Defense:
As AI continues to advance, we may witness the emergence of autonomous cyber defense systems that can detect, analyze, and respond to threats without human intervention.
Collaborative Threat Intelligence:
Machine learning can facilitate the sharing of threat intelligence across organizations, enabling a collective defense approach against cyber threats.
The rapid evolution of cyber threats necessitates a corresponding evolution in cybersecurity strategies. Machine learning, a subset of artificial intelligence, is revolutionizing the field by providing proactive and adaptive solutions to defend against evolving cyber threats. From detecting anomalies and behavioral patterns to real-time response and vulnerability management, machine learning offers a multifaceted approach to safeguarding digital assets.
While challenges remain, ongoing research and advancements in machine learning techniques promise to address these issues and unlock even greater potential for AI in cybersecurity. As organizations continue to embrace these technologies, the symbiotic relationship between AI and cybersecurity will likely play a pivotal role in creating a safer digital ecosystem for individuals and businesses alike.
Read This Now – AI Creativity: The Intersection of Artificial Intelligence and Artistic Expression
Hello sparerun.com webmaster, Your posts are always well presented.